As we move into a new decade, it’s safe to say that “real-time data” was one of the buzzwords of the 2000’s and 2010’s. In parking, when people talk about real time data, they usually refer to real time data from stationary sensors. Initially, stationary real time sensors played a big role in traffic information - but those days are long gone. Today’s traffic information is based on vehicle and smartphone sensor data and predictions. Stationary sensors are marginalised. Will the same happen in parking information?
But is it always best to rely purely on real-time data?
In parking, one source of on-street real-time availability data is stationary sensors (either electro-magnetic or infra-red), which are installed in the middle of a space to sense if there is a vehicle on top of it occupying the space. However, if just one sensor breaks in a group of 5, the data for all 5 is reported inaccurately due to aggregation across all 5 spaces. There is also the cost of installing and maintaining sensors in the street environment that can be prohibitive. In the UK, Westminster City Council have turned off their much-lauded smart parking street sensor scheme, though it is unclear if this decision was due to maintenance costs or another factor. San Francisco turned off its stationary parking sensors a while ago.
Another question is whether “real-time” is actually real-time? In reality, there is a minimum lag-time of at least 1 minute between the source system reporting an available space to the cloud, the data linking with a digital parking services provider such as Parkopedia, and then getting that data into the car system of connected vehicles in the vicinity who are searching for spaces at that time. In most cases the latency will be significantly higher, so real time data from sensors is actually historical information.
So what are the other options for parking availability data?
- One potential source is car sensor data. However, whilst this data is perceived as accurate in general, connected vehicle data has the same time lag issues as with stationary sensor data, and additionally many suffer from GPS error. With a “parking event” in an urban environment (where parking pressure occurs), the GPS location is generally accurate to 1-2 metres. As the urban environment becomes increasingly built up with tall buildings and skyscrapers, line of sight to the GPS satellite is increasingly compromised, meaning the data inaccuracy and error count will only increase. Inside a city where errors can be up to dozens of metres, the result is car sensor data that reports a parked location as being in a different road section or an adjoining road. In testing with some of our automotive partners, we’ve seen results showing open parking spaces “off-road” and in solid buildings!
- Transactions data is another option. However this too is flawed if used as the sole source of truth. In many cities, there are multi-space meters that cover several streets, which means you can’t tell which (part of a) street has actually been parked on, and therefore where spaces are available. Even with a system able to identify individual spaces, the data reported is often not fully correct, as drivers may over-pay, resulting in a free space that is not reported as such, or under-pay, where the space is still occupied even after the paid time-limit has expired. Transactions data also doesn’t take into account anyone with a permit (eg residents or disable badge holders) who are exempt from the payment, but may still occupy a paid parking space nor does transaction data cover hours of operations when parking is free.
At Parkopedia, we fuse multiple data sources - static parking data, ground surveys, real-time parking sensors, car sensors, transactions and imagery to overcome potential errors or gaps, and combine these with machine learning models, to provide predicted parking availability. With a combination of historical behaviour patterns and real-time data from stationary and mobile sensors, these predictions are able to produce an accurate representation of the current situation (which is effectively a prediction based on “real time” data that is usually already a few minutes old).
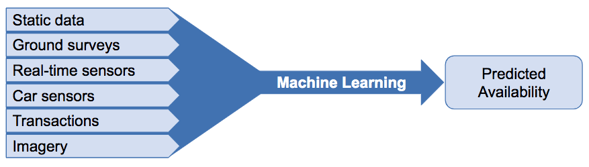
This approach offers Parkopedia’s B2B customers an industry-leading solution that they can provide to their drivers with confidence. Parkopedia predictions are rigorously tested, as we run a continual programme of ground surveys to manually collect and check all relevant information including the accuracy of our prediction models globally.
In tests using transactions as the sole data source, Parkopedia’s predictions models based on fusing multiple data sources excluding transactions perform up to 2x better, and we see an additional 15-20% improvement in accuracy prediction when transactions are included.
If you would like to find out more about our Probabilistic Forecasting, or fancy a deep dive into the Theories of Probabilities and the Brier Score, please get in touch!
You can read more Parkopedia blogs here.